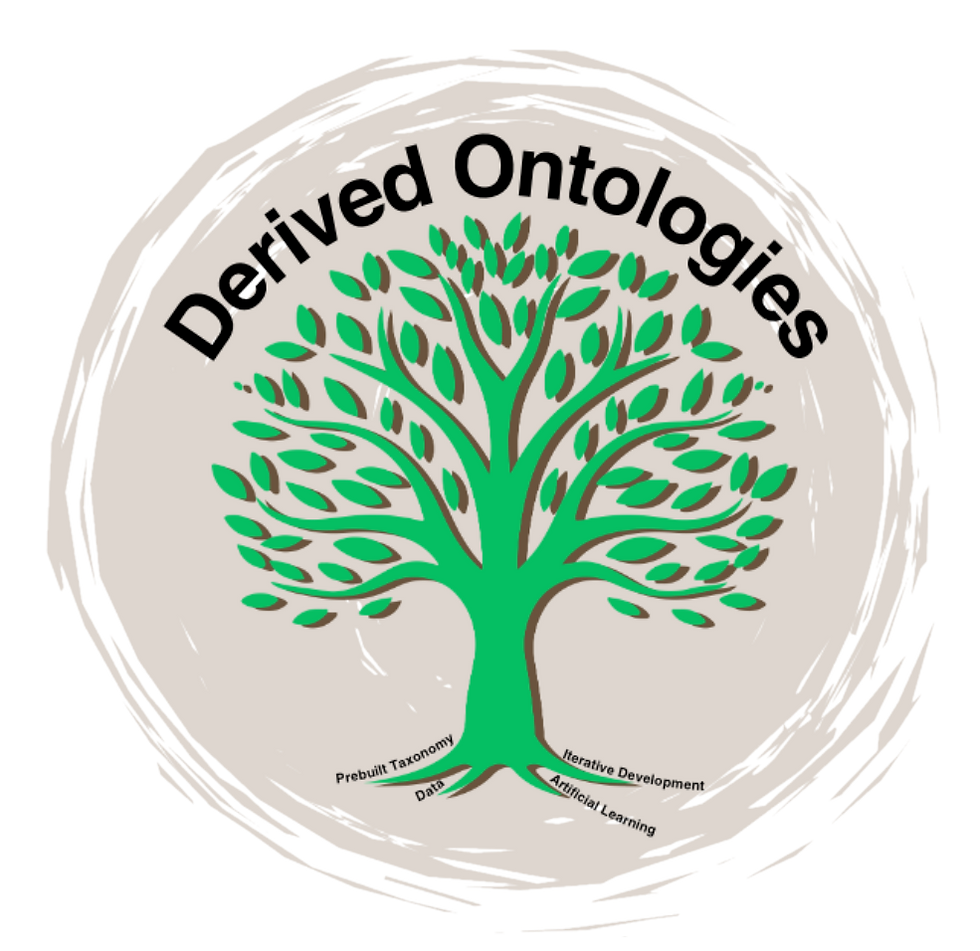
As you or your client’s company grows, I am sure you have noticed the increase in data and documents. These things can quickly bog down your system and users. Without an effective knowledge management system, workers and processes quickly become inefficient. If your users cannot access the information they need, how are they supposed to complete the work to which they are assigned?
This problem can only get worse, as users who are unable to find the information they are looking for, decide to just recreate the content. Unfortunately, this only worsens the inconsistent and inaccessible data problem as now there are multiple versions of the same information floating around, each labeled differently and living in different silos.
How can your company overcome this serious issue? Is it too late to fix the data problem? (This would be a very disheartening article if I were to say yes…) Thankfully, there are solutions on the market today that address your very needs! The answer comes in the form of a knowledge management tool known as derived ontologies.
What Are Derived Ontologies?
Ontologies are structured frameworks that represent knowledge in a particular domain and their relationships to one another, allowing for communication across people and computers.
Prebuilt ontologies, unfortunately, come missing some of the key terms and relationships unique to your business. The missing terms come as no surprise, as each organization has its niche, and prebuilt ontologies only serve as an 80% solution. One should expect to need to make minor changes to fit each company’s data perfectly. Once these modifications have been made, the organization ends up with a derived ontology.
A derived ontology is simply an ontology that has been modified by extracting and integrating data and concepts from the existing ontology model, or even from outside data sources. This derivative process typically involves indexing, analyzing, and adding new terms from a variety of data sources. The resulting ontology provides more focused, tailored representations of the key terms and relationships with one another.
The process of creating derived ontologies could be broken down into 5 easy steps, which we will outline in detail below.
1. Defining Knowledge Management Goals
2. Assessing Source Data and its Reliability
3. Selecting Appropriate Taxonomies
4. The Iterative Development of Derived Ontologies
5. Continuous Improvement
Defining Knowledge Management Goals
Obviously, to get a high-quality result, one must fully understand the issue and the goal. If we don’t know where we are going, how can we possibly get there? Who will use this knowledge? Why will it matter? What value will be created for the enterprise? All of these are good questions to ask before starting down the knowledge management path.
Assessing Source Data and its Reliability
Assuming that your company is interested in knowledge management also assumes that there is a corpus of documents and data already in your company. But not all data is good data, nor is every document reliable. Before we can effectively analyze and manage the data, we must understand the reliability of it. Taking polluted data will turn any effective knowledge management initiative into a massive waste of time.
Selecting Appropriate Taxonomies
After we understand exactly what we hope to accomplish and with what data we want to use, we must select appropriate taxonomies to jumpstart our knowledge management project. If we are expecting to create a derived ontology, we must start from a solid beginning. There are hundreds of different kinds of taxonomies available to use, and matching the right taxonomy to the right data is paramount for success. It allows us to extract meaningful analytics and results from our data.
The Iterative Development of Derived Ontologies
Now that the basic setup steps have been accomplished, it is time to begin working on the focal point of our knowledge management: the derived ontologies. While you can accomplish a lot with out-of-the-box taxonomies coming together in ontological form for your knowledge management, we want to take it a step further and create a top-notch knowledge management system. This iterative process really has three steps, involving both man and machine in the refining.
First, we will need to apply the prebuilt taxonomies to our corpus of documents. Starting here allows us to find, and eventually fill, all the gaps that may arise in the prebuilt taxonomies. After indexing with our out-of-the-box taxonomies which we have connected into one ontology, domain experts will need to review the results. During this review process, they can identify new terms and phrases that do not currently exist in the ontology. Thirdly, these new terms will be added to the ontology, and we will re-index the documents.
As this is an iterative process, we will need to repeat these three steps multiple times to increase accuracy and ensure completeness.
Continuous Improvement
Building on our last step of iterative development, maintaining a derived ontology is going to be an ongoing project. As times change, documentation increases, and new concepts are added to our business world, these modifications will need to be added to our derived ontology. There are two parts to this continuous improvement: First, as general factions of our businesses change, we can add generalized terms via our prebuilt taxonomy seller. Second, as our individual business changes or adds concepts and processes, we can add those new concepts and relationships following the iterative process outlined earlier.
As a closing thought, in this day and age, data is king. The ability to effectively manage and utilize information has the possibility of setting businesses apart from their competitors. Leveraging high-quality derived ontologies can allow businesses to turn vast amounts of data into valuable knowledge. Valuable knowledge then in turn drives informed decision-making and strategic growth.
Comments