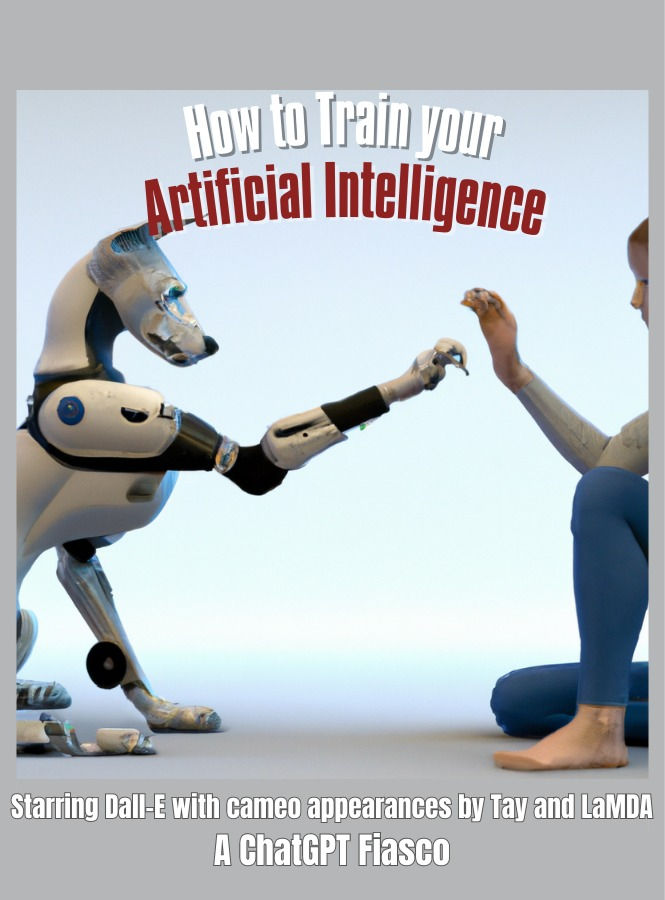
Microsoft's Bing is an emotionally manipulative liar. AI chatbot "Tay" turned racist in less than a day. ChatGPT is sexist. Headlines like these are becoming more and more frequent as AI chat bots become more popular. Their freakish ability to mimic natural human language is fueled by the unsupervised learning and deep learning strategies employed. The massive amounts of unstructured and unregulated text being fed to these engines from sources around the internet allow the bots to synthesize human speech patterns.
Unfortunately, human speech on the internet has always had issues with things like racism, manipulation, sexism, and so on. And chatbots trained on internet text found and repeated these negative conversational patterns rather quickly. Suddenly, many companies started scrambling to retrain their AI bots to not mimic the biased and disgusting parts of text found in their massive amounts of unregulated training data. The fine-tuning process includes techniques such as Reinforcement Learning from Human Feedback (RLHF), specifically designed to manually minimize any biased and harmful outputs. But this process is tedious and some question the ethics of the processes employed. These problems may be largely avoidable, however, if the proper tools are employed prior to the initiation of the machine learning process.
AI for the Enterprise
The benefit of training a model on massive amounts of data is the accuracy of the mimicry. Models can quickly understand not only individual words, but concepts and relationships between words. The more text, the more human sounding the content. But there is still the problem of helping the model understand what relevant content is and what it is not. Instead of hand-training the bad traits out of a model and spending countless hours and resources, why not address the issue before it presents itself?
Instead of waiting for problems to arise from this unsupervised learning and putting in hours of manpower to remedy the issues, organizations can use taxonomies/metadata models to provide the AI models with a structured starting point. When an artificial intelligence model is first created, its knowledge base is empty. And just like a small child, the engineers need to help it begin to build up that knowledge. The main difference between supervised machine learning and unsupervised machine learning is the process of exposing the AI model to the data which allows them to learn. Unsupervised learning models involve exposing the model to a vast amount of data and giving it no guidance other than to learn patterns and connections within the given data. Supervised learning provides the model with a starting point –– with parameters.
As you can imagine, if a model is given parameters, or a base of specified knowledge with relationships, the content they generate is more likely to come back with expected responses. In the case of these large AI chatbots such as ChatGPT and Microsoft Bing Chat, which were trained on the internet with relatively little guidance, their responses can often be unexpected. Now think about your own company. If you were to have an AI-powered chat bot there to help with daily business activities, imagine the difference that it would make to first train the model off a relevant set of hierarchical terms like those found within a curated, domain-specific taxonomy/metadata model. After getting this training data set, the AI model can then be sent into the depths of your company's documents and allowed to continue to learn to make connections and inferences off data, but armed with a set pattern and therefore a deeper understanding of relevant content and the appropriate responses.
Clearly, as with any training style, taxonomies do not completely remove the need for a human touch. There is still a time and place for RLHF methods. However, the time and resources spent on that reinforcement learning processes can be greatly reduced by addressing the potential incorrect assumptions before the model teaches those to itself.
As more and more headlines get released and new chat bots enter the business world, it can be concerning as a company to consider training their own AI for fears of ending up with yet another racist, sexist, split-personality bot. But there are ways to not only address these fears, but also cut down significantly on the time and costs behind training an AI model. In fact, there is a high probability that training and employing a LLM within your business will be extremely beneficial to the point where it outweighs the risks. Furthermore, you don't even have to create your own taxonomy from scratch! Here at WAND, our expert taxonomists create curated, prebuilt taxonomies which you can employ in training your AI model with just a few clicks and not only save time and money, but also reduce the likelihood that you will end up with a Skynet-type robot wreaking havoc in your company.
You can read the articles mentioned above by following the included links:
Microsoft's Bing is an emotionally manipulative liar, and people love it: https://www.theverge.com/2023/2/15/23599072/microsoft-ai-bing-personality-conversations-spy-employees-webcams
Twitter taught Microsoft's AI chatbot to be a racist asshole in less than a day: https://www.theverge.com/2016/3/24/11297050/tay-microsoft-chatbot-racist
Is ChatGPT Sexist?: https://www.forbes.com/sites/tomaspremuzic/2023/02/14/is-chatgpt-sexist/?sh=773aad946b6b
We Got a Psychotherapist to Examine the Bing AI's Bizarre Behavior | There's some strange psychology afoot here.: https://futurism.com/psychotherapist-bing-ai
ความคิดเห็น